By Natasha Bhatt / April 26, 2024
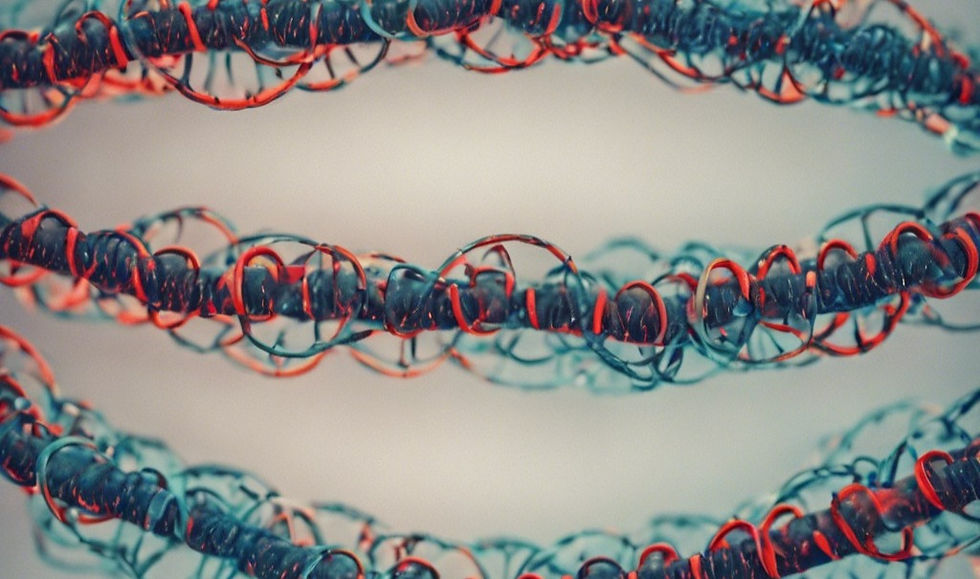
In the present-day digital era, artificial intelligence has been used in numerous discoveries, but with these innovations, the transparency of AI-related work is just as crucial. A neural network, which replicates the human brain, is an AI-invented work that is not opaque to the inner workings of machine learning. However, computer scientists from New York University made a ground-breaking discovery, breaking the secrecy of neural networks. This development holds promise in deciphering biological processes of genomics, with RNA splicing–which plays an important role in transferring information from DNA to functional RNA and protein products–being its primary focus.
Under the direction of Professor Oded Regev, their research and model created provides insights into the underlying molecular pathways in addition to reliably predicting splicing results. The researchers used extensive experiments to verify their predictions using the model. The model illustrates how certain RNA structural characteristics, such as the shape of a hairpin forming, affects the splicing efficiency. The model gives priority to the interpretability factor.
These findings from the NYU Institute enhance the field of RNA biology and its uses in industry and medicine. Gaining insight into the intricate details of RNA splicing enables the development of specific treatment approaches which were previously undiscovered. It provides the previously unheard clarity and transparency into the molecular process of life, a major advancement in genomics and machine learning.
To read more about this research the model created by the New York Universities computer scientist, visit: https://www.pnas.org/doi/10.1073/pnas.2221165120
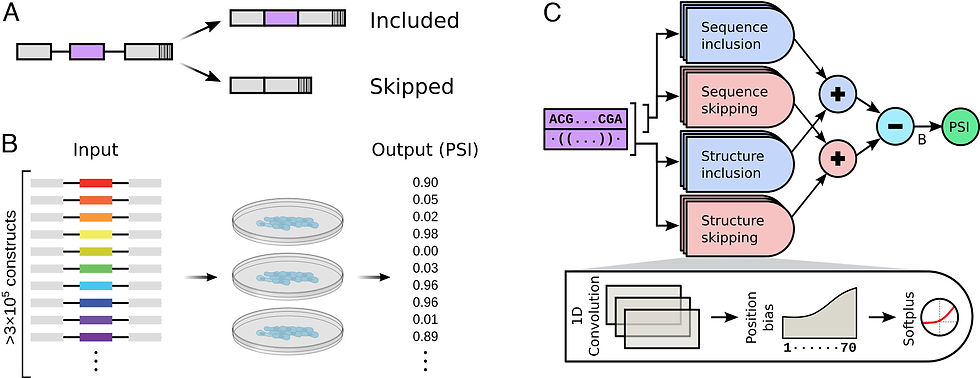
Glimpse Into NYU’s Research
Citations:
Communications, N. W. (2023, October 6). Researchers create a neural network for genomics-one that explains how it achieves accurate predictions. NYU. https://www.nyu.edu/about/news-publications/news/2023/october/researchers-create-a-neural-network-for-genomics-one-that-explai.html#:~:text=%E2%80%9CBy%20harnessing%20a%20new%20approach,it%20arrives%20at%20its%20predictions.%E2%80%9D
Liao, S. E., Sudarshan, M., & Regev , O. (2023, October 5). Deciphering RNA splicing logic with interpretable machine ... Deciphering RNA splicing logic with interpretable machine learning. https://www.pnas.org/doi/10.1073/pnas.2221165120
Comments